How will AI revolutionize data & analytics?
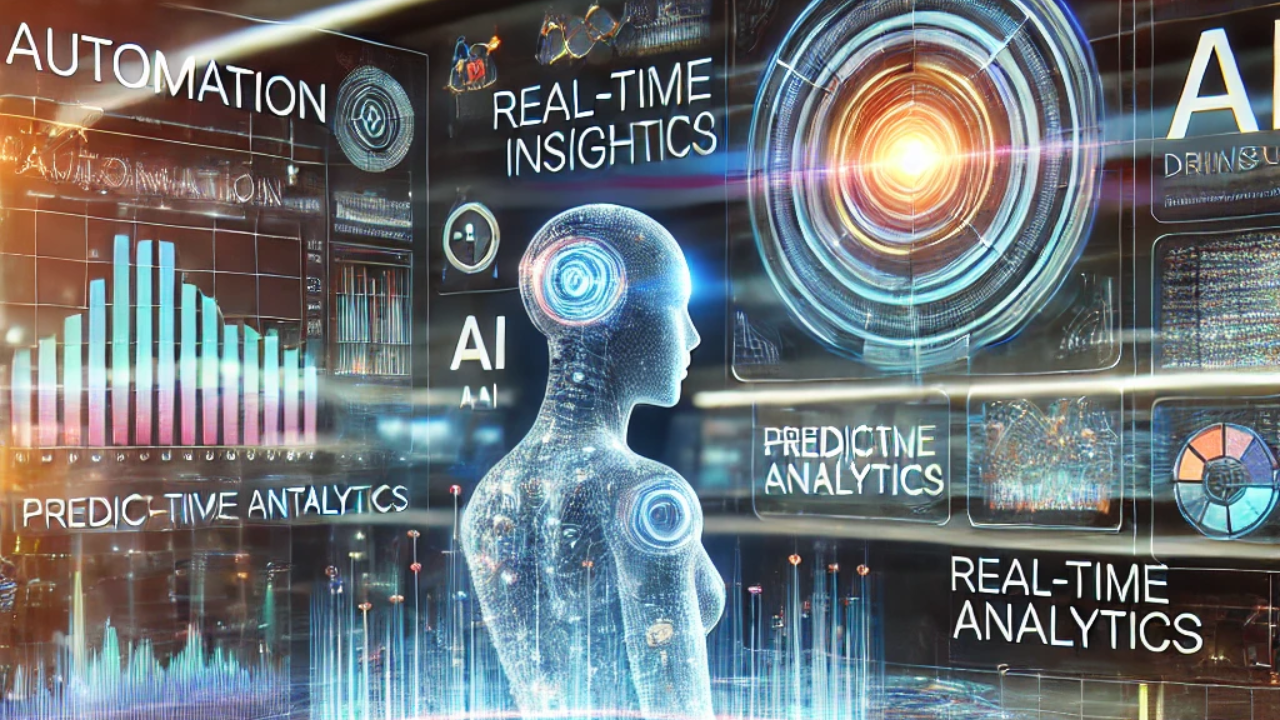
Introduction
AI is the cornerstone pillar of many industries and with this relatively new area in terms of its maturity, AI is being explored in multiple industries and functional areas. Similarly, AI has had a huge impact on how data analytics industry is operated, perceived, and disrupted. Data Analytics is considered to be a core capability of an organization with its capabilities being utilized across multiple teams. The data analytics team helps in two main things:
- making sense of the data
- create automated tools and frameworks to help structure the unstructured
In my opinion, AI will not change these fundamentals, but rather transform them. AI will demand transformation in skillset from being code or tool driven to more "understanding the business problem" driven. It will also change the way data teams are perceived - data teams will always be required, however, they will need to do one more important bit:
- empower other teams to make data-driven decisions
Once empowered, data teams will move on - to newer and better business problems. Spending time on running the operations and sustaining the solutions will reduce or will be contained to only more complex data solutions.
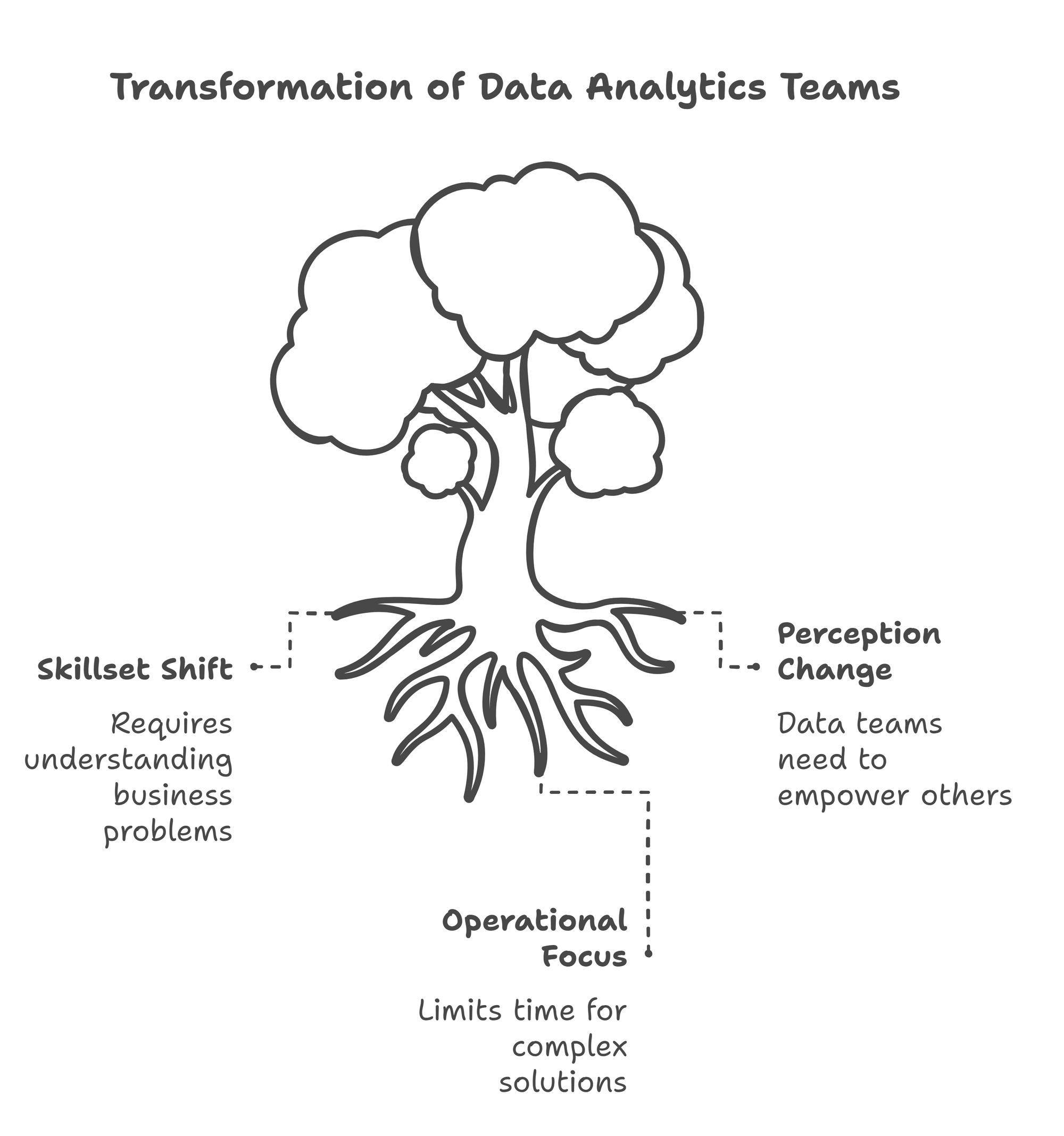
The current landscape of data analytics
As mentioned in the section before, data analytics industry is being transformed at a high scale. At the same time, I believe it is important that we do not over-estimate the penetration of AI in analytics.
AI has not been disrupting the data industry now – its an old news. AI encompasses machine learning - and machine learning has sustainably taken over the ways use cases are solved over the years. However, leaders are now talking about AI taking over their teams in a panicky way - which creates unrealistic picture and wrong estimations. What needs to be truly assessed is - h0w much is the requirement for net new penetration and is it even relevant?
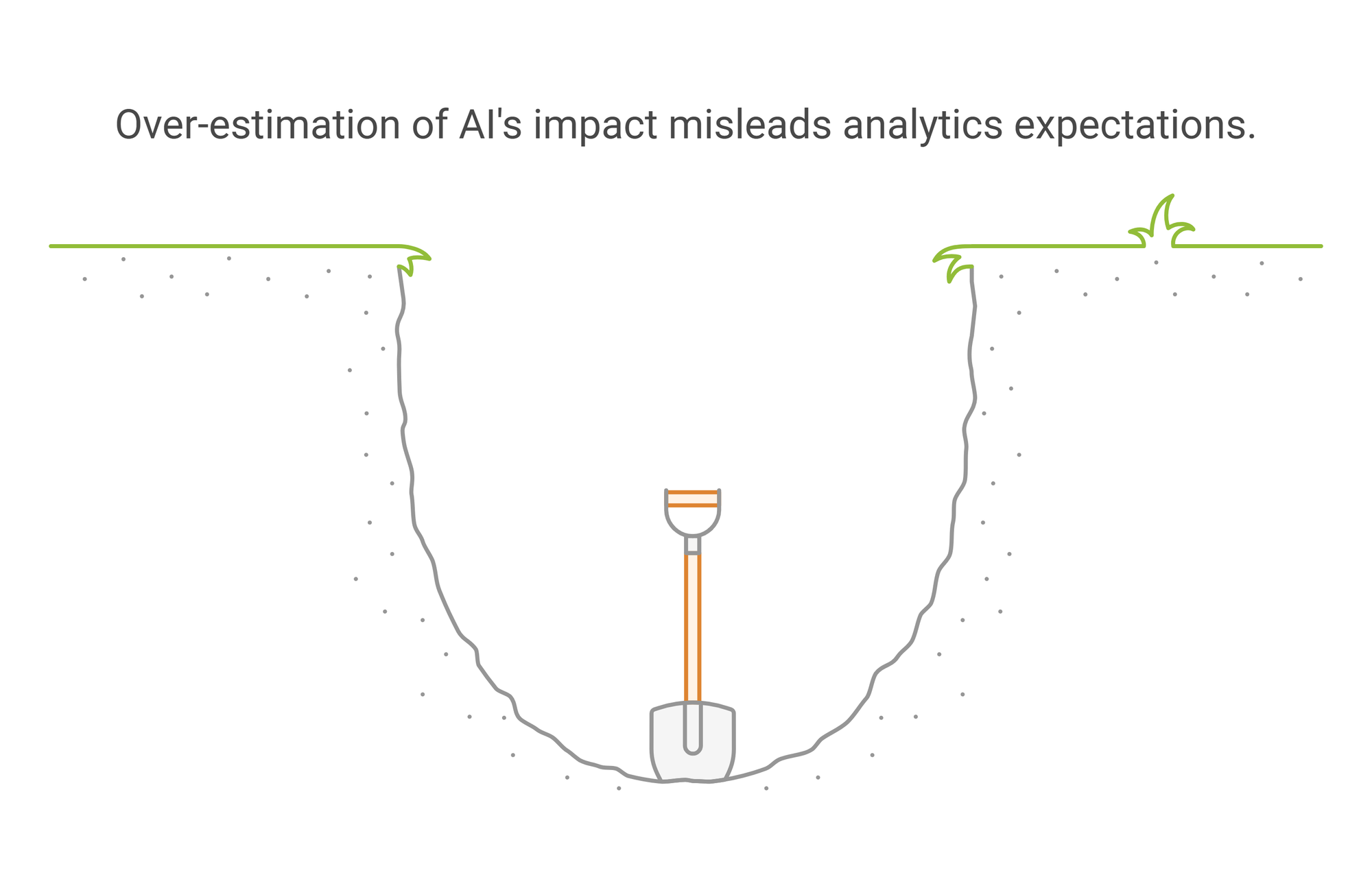
Some of the examples of use cases which are solved through AI (machine learning) are provided below. These use cases will continue to be solved through ML, with an added opportunity to create smarter and more intelligent workflows. For instance, these use cases can potentially be coded by a data analyst and not just a trained ML engineer given the range of different LLM solutions helping us to code.
Insights into consumer behaviour: retrospective analysis can give us insights into the patterns of consumer buying, spending, interacting and indulging. This has shaped a lot of the use cases across healthcare, retail, CPG, real estate and other industries.
ML use cases: AI is used to solve for supervised and unsupervised learning use cases such as classification analysis (detection of fraud, segmentation of customers, etc.), regression analysis (prediction of stocks, sales, inventory), clustering analysis (clustering and segmentation of customers, detection of underlying patterns, etc.) and even forecasting.
Automation and efficiency gains: AI is continuously used to automate workflows and also reduce human intervention.
In all, the usage of AI in data analytics is not new - it is used across business teams for many adhoc analytics and also across IT teams for end to end automation of many use cases.
However, there is one area in analytics which I strongly believe is relatively less mature and will (should) face significant disruption given the AI revolution across market - and that area is business intelligence. Data analysts today are being consumed into data to day analysis and preparation of dashboards for adhoc stakeholder requests. Ultimately, BI and reporting is an integral part of day to day tasks of a data analyst. According to my experience:
- most of the dashboards are one-offs and are not utilized regularly
- the ones utilized regularly do not provide a comprehensive picture and need to go through multiple rounds of enhancements
- even if there is a robust solution for BI, each stakeholder's demands are different rendering the relevance of solution very subjective in nature
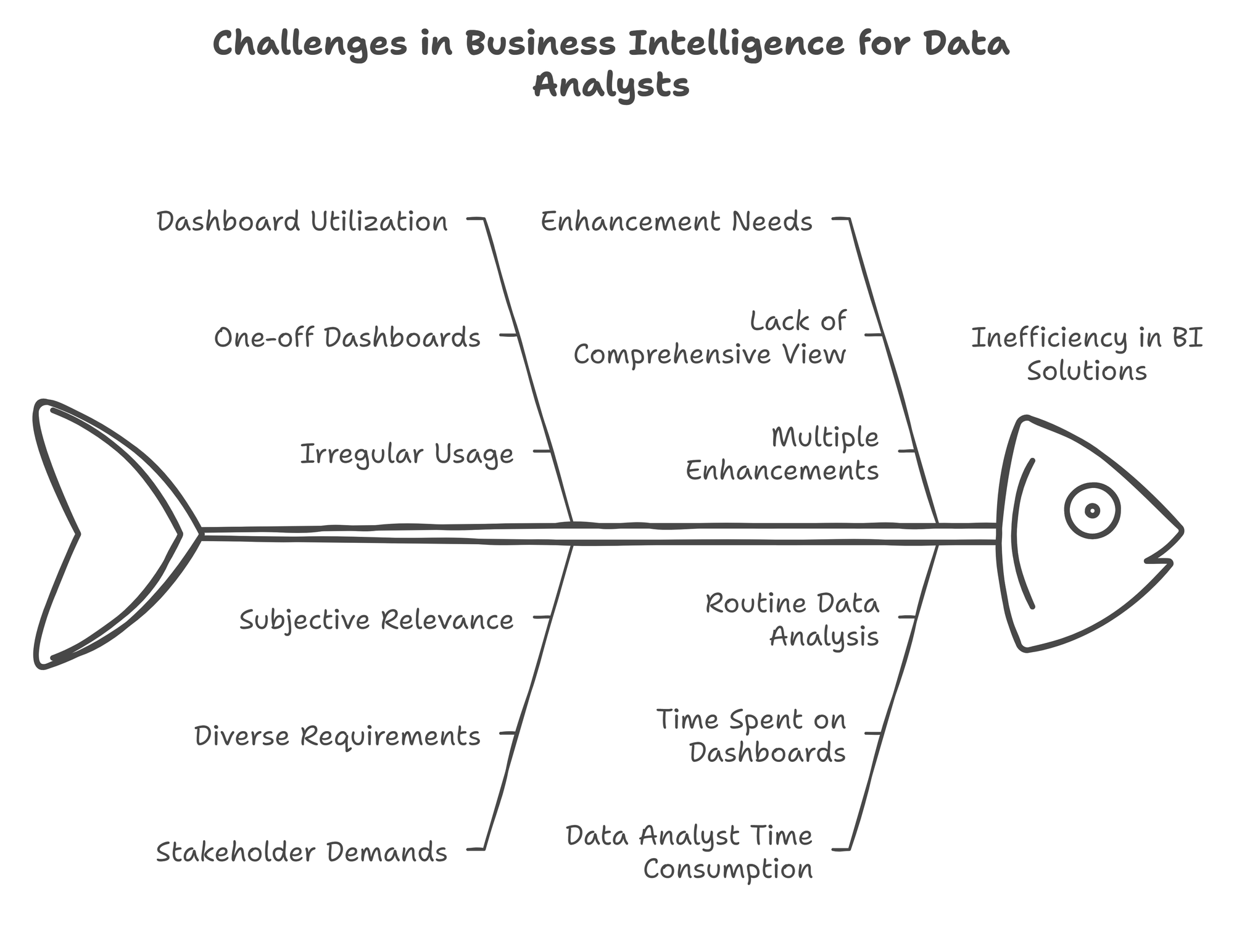
According to Brownlow (2023), dashboards are not dead but are dying and it is also transforming the day to day tasks and operations of the analytics professionals.
One of the snippets from her blog on medium:

AI analytics is a field that will help us solve for this. This is a field which will empower the business stakeholders to derive insights directly, reduce the time spent by data analysts on these tedious tasks and optimize the strength of the analytics teams - as these kinds of reports would be fully automated in future.
The rise of AI in analytics
A few years down the line, AI's role in analytics will be realized even further. Tools such as ThoughtSpot, Pyramid Analytics, Luzmo, Tealium are being accepted due to their cutting edge Generative BI aspects.
According to Gartner (2024) –
"GenBI features in ABI tools are novel, unproven and untested in production environments. Expectations of these capabilities outpace the reality of execution".
I strongly believe that GenBI solutions will quickly mature in production environment and scalability will be the on the rise. In fact, as per the Gartner's magic quadrant of 2024, ThoughtSpot (which is one of the leading GenBI solutions provider) was named as a leader across the overarching BI tools and solutions.
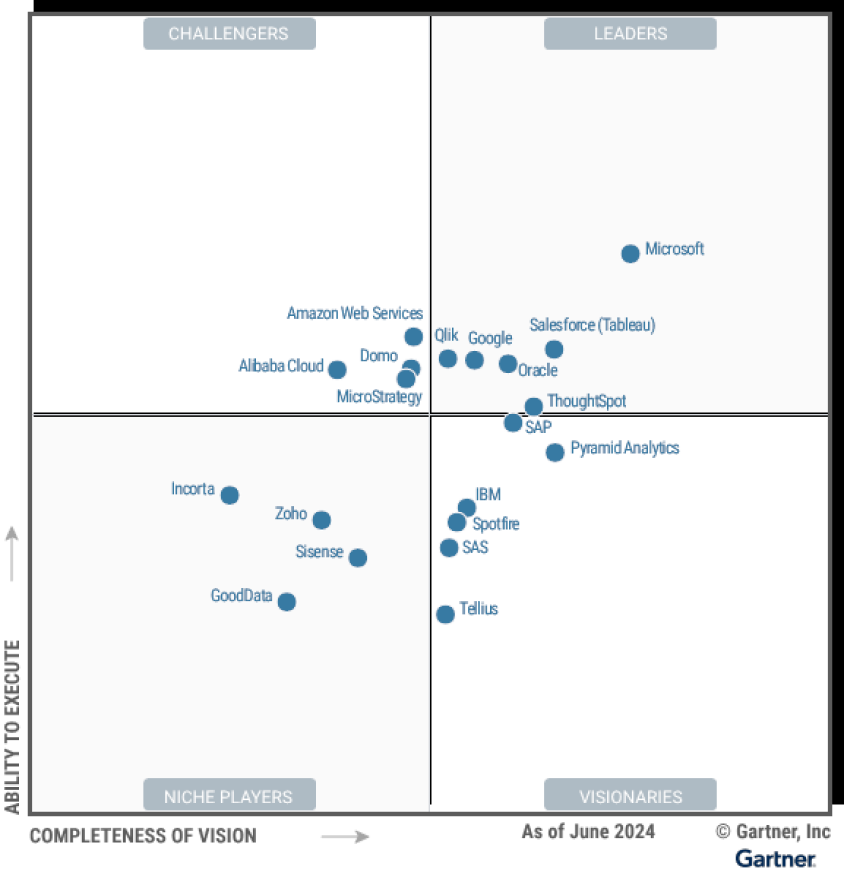
Tools such as ThoughtSpot will give rise to self-serve aspect and will instil trust among business stakeholders about the reliability of the insights. In future, what the data and analytics team will do is to - ensure that the trust is maintained by:
- democratizing data and capabilities
- making sure that the right data products are created and available as the source for the GenBI solutions
- helping to dig deeper across more advanced analytics to support business stakeholders
Below, I have created a sample framework to assess any GenBI solution's capability in terms of its integration into an organization and scalability.
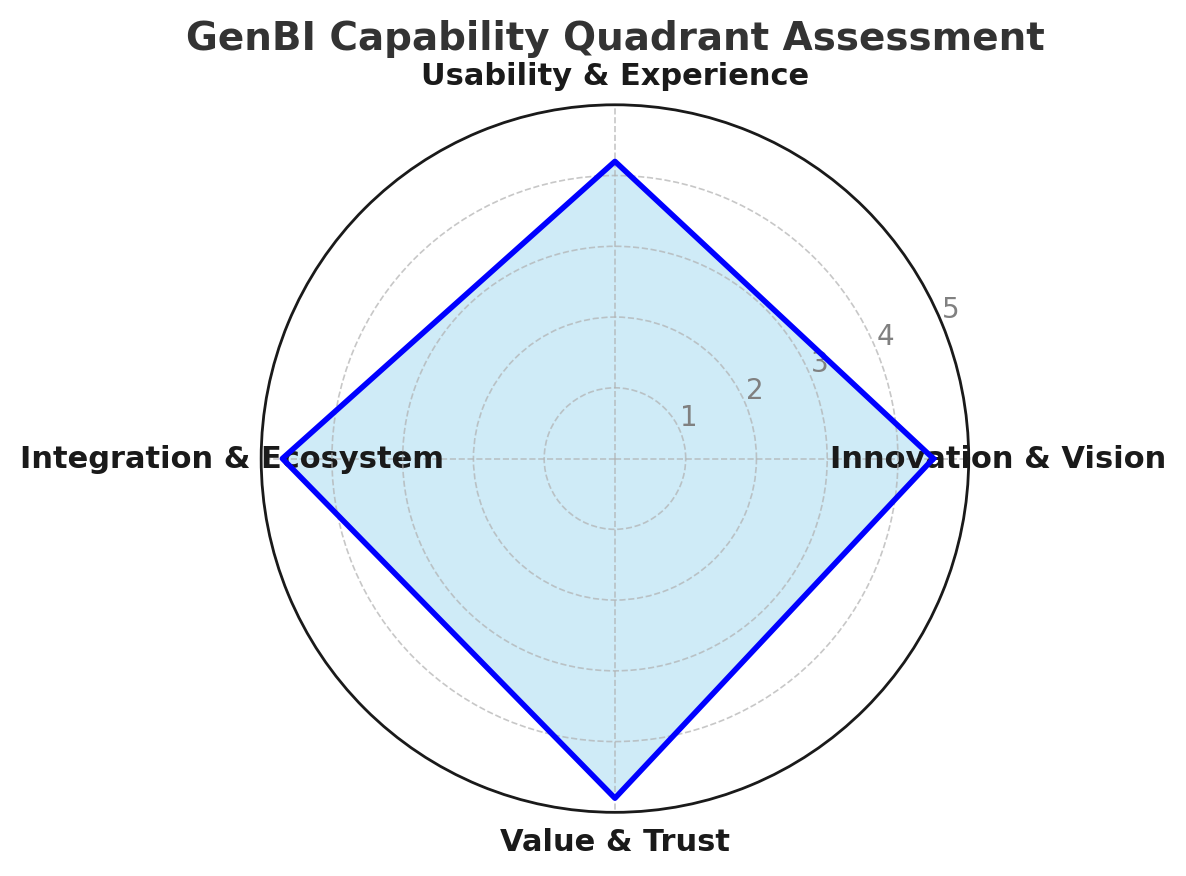
In my opinion, this change will not happen overnight. The acceptance of the GenBI solution depends on many factors and it will be a cycle that includes - hesitation at first, then there will be a dual acceptance horizon (GenBI being used only for specific tasks), then a maturity curve and finally - the organizations will accept GenBI fully and retire the customary BI solutions eventually. Below visual depicts this idea:
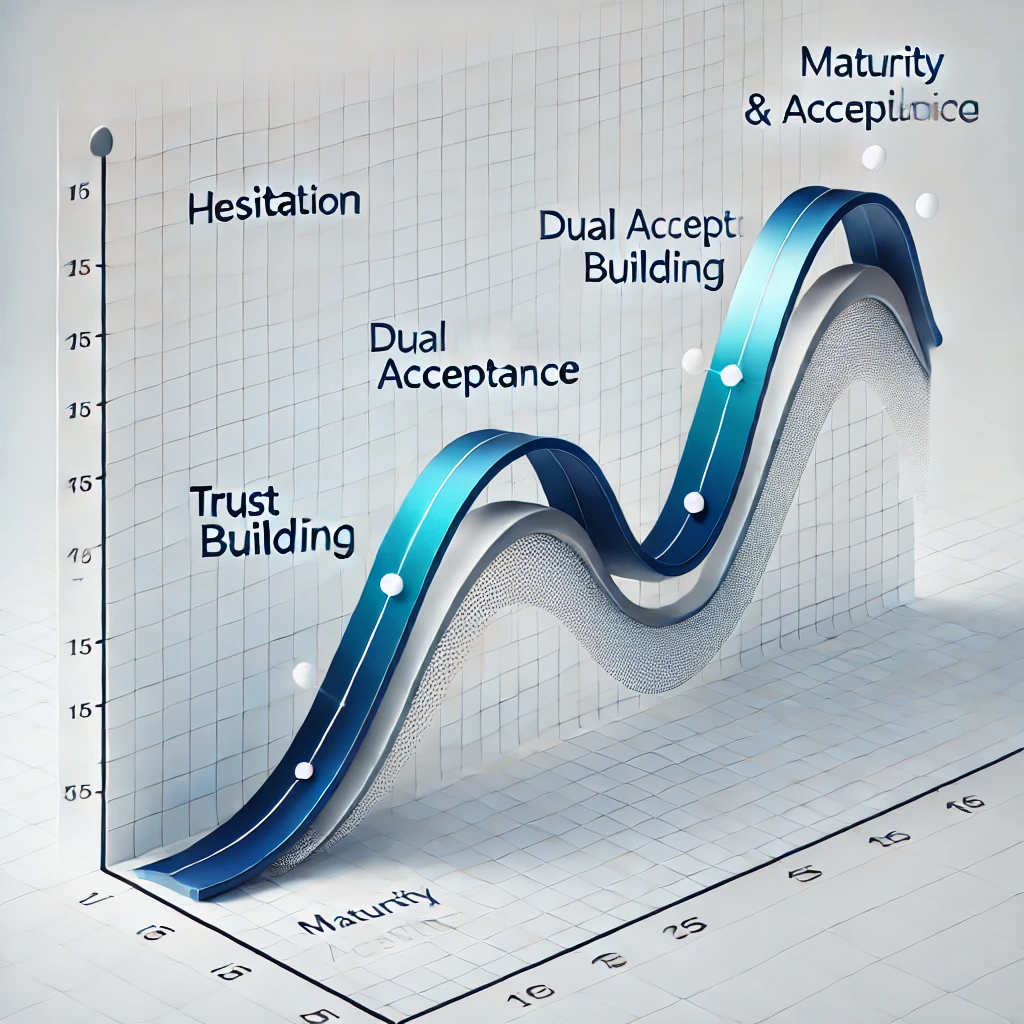
GenBI will not replace analysts - it will empower them to work on more advanced problems and will even make that part easier for them. Use cases such as code generation, creating visualizations on the go, writing machine learning codes and even creating end to end data modelling workflows will empower data analysts to thinking strategically rather than getting bogged down by the manual aspect of "coding solutions".
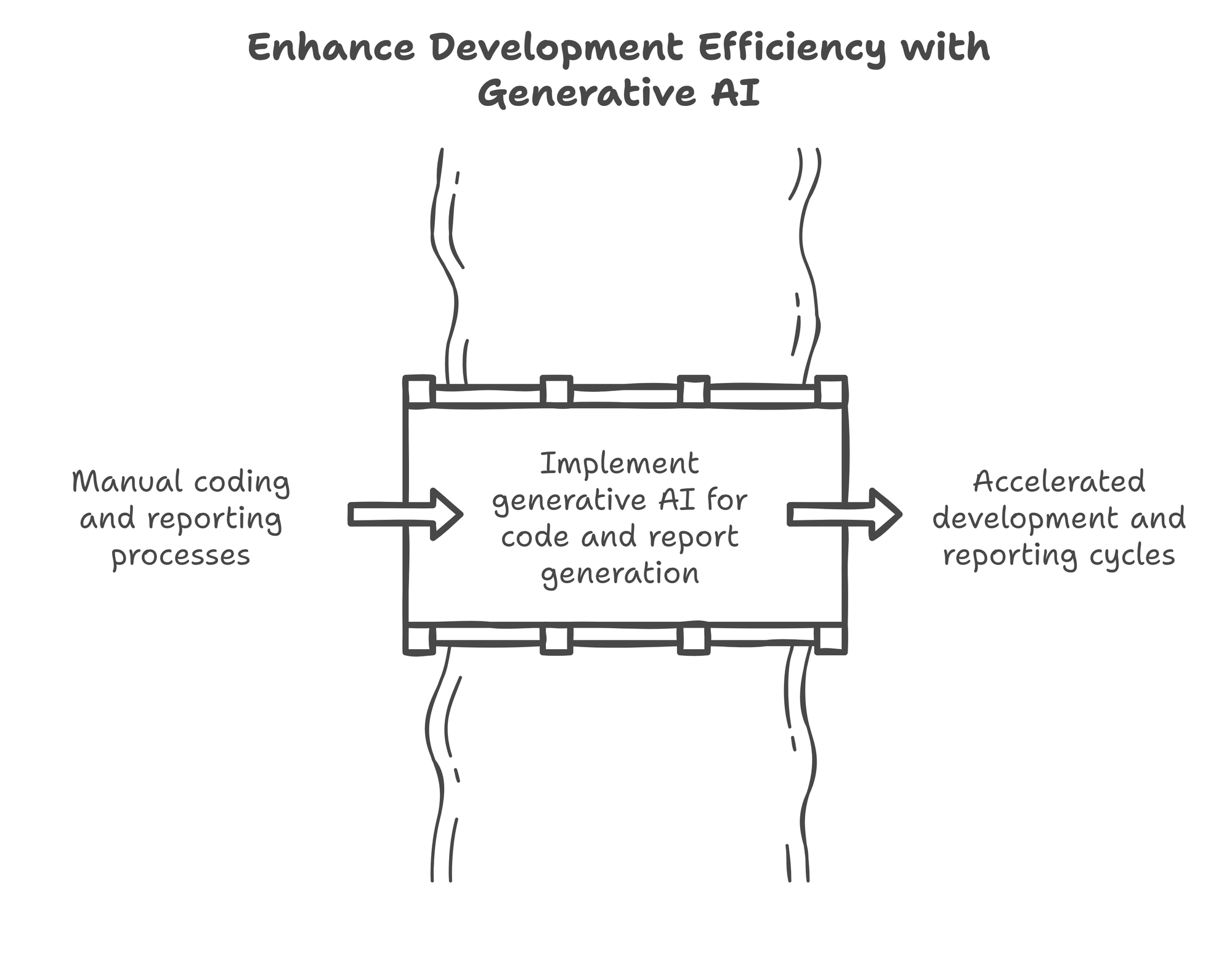
The rise of citizen data scientists
In the GenBI era, the role of citizen data scientists is no longer aspirational but essential. These individuals, empowered by generative AI and intuitive BI platforms bridge the gap between technical expertise and business acumen.
Key drivers:
- Accessibility: Tools now leverage natural language and search-driven analytics, enabling non-technical users to derive insights effortlessly.
- Efficiency: Reduced dependency on traditional BI teams accelerates decision-making.
- Scalability: Democratizing data capabilities ensures that insights flow across all organizational levels.
Strategic Imperative:
Organizations must foster this evolution by investing in training, embedding trust and governance into their GenBI solutions, and encouraging a culture of data-driven innovation.
The result? Faster decisions, empowered teams, and a competitive edge in an increasingly data-centric world. Citizen data scientists are the linchpin of this transformation.
usiness stakeholders of 2030s will soon become the data analysts of 2020s.
How to prepare yourself for this revolution?
One aspect of being ready is mental preparation.
The sooner we accept, the better it is. Revolutions have always prepared humans generically - be it the IT revolution in 2000s or the computers in 1990s. Data Analysts need to be focused more on strategy, helping to monitor data literacy, ensuring that they empower others successfully. Most important bit is - not feeling insecure about sharing your knowledge with others. A lot of times, people think that if they share their knowledge, they will be replaced. However, the ones who share knowledge are the ones who are trusted.
Second aspect is skillset.
I think that the generic data analysts who know what visualizations to create and when, and which models to use for which problems will always be relevant. Their knowledge will be rather augmented by the AI solutions. They will be able to do their jobs easily. So the important bit as of now is to stay up to date as per the market solutions, tools and trends.
It is important to have a POV.
When the time comes, it is important to have a point of view on the right solutions for your use cases. Having a sense of frameworks which can help you assess the right tools for your team will be a key. Whether the solutions are scalable, flexible and have the robust security in place - will be important to evaluate.
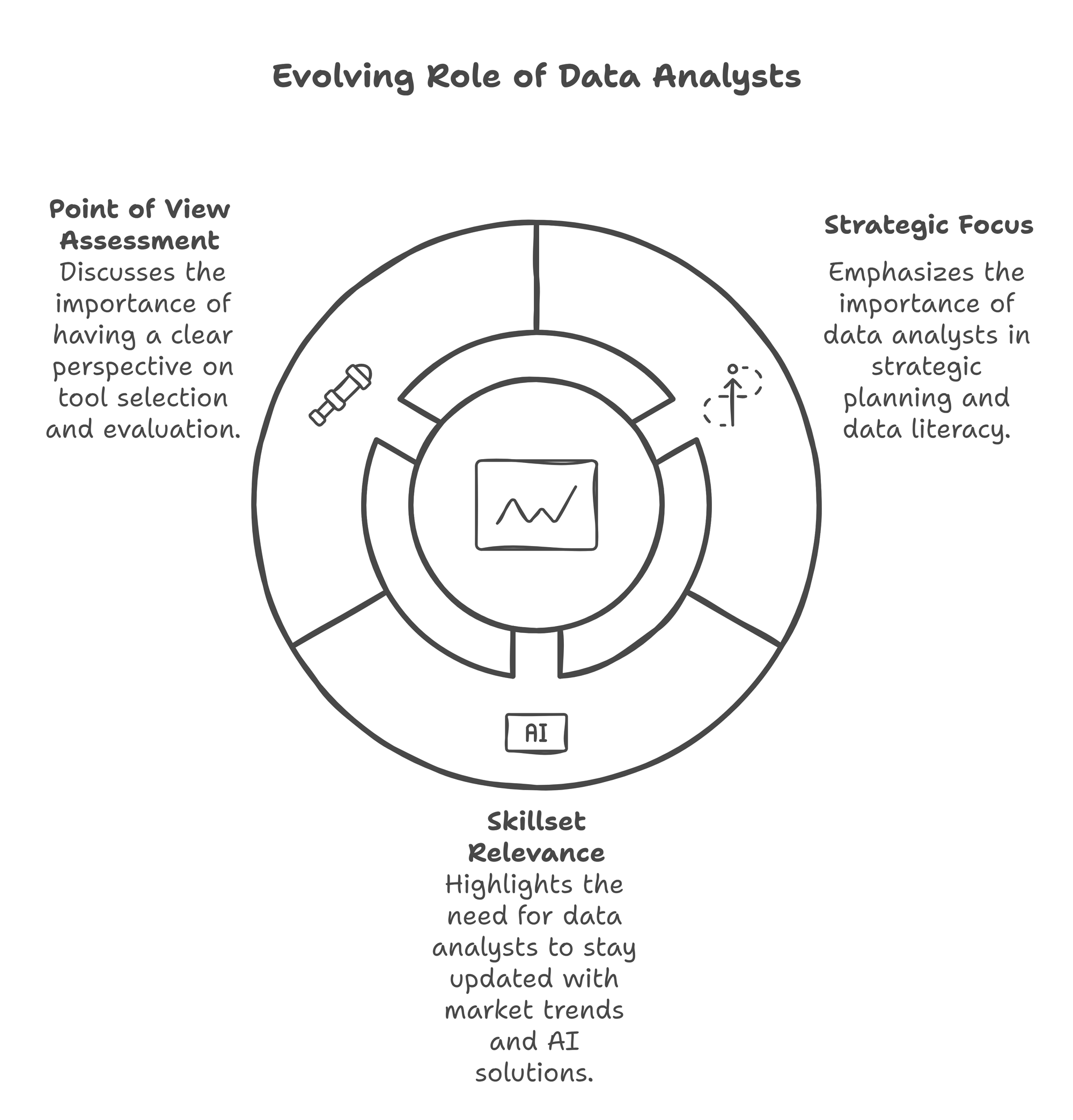
Conclusion
AI is already being to solve various use cases already - it is important for us to understand its penetration into our orgs to evaluate the "unmet needs". However, AI will definitely penetrate data analytics industry further and it will do so deeply. Data Analysts will need to prepare themselves, stay up to date with the industry tools and trends, and think strategically. Business stakeholders will need to accept the era of citizen data scientists and will have to do a lot of tasks themselves instead of relying on data analysts. Data democratization and literacy will be the pinnacle of the organization's vision in the next 10 years.
References
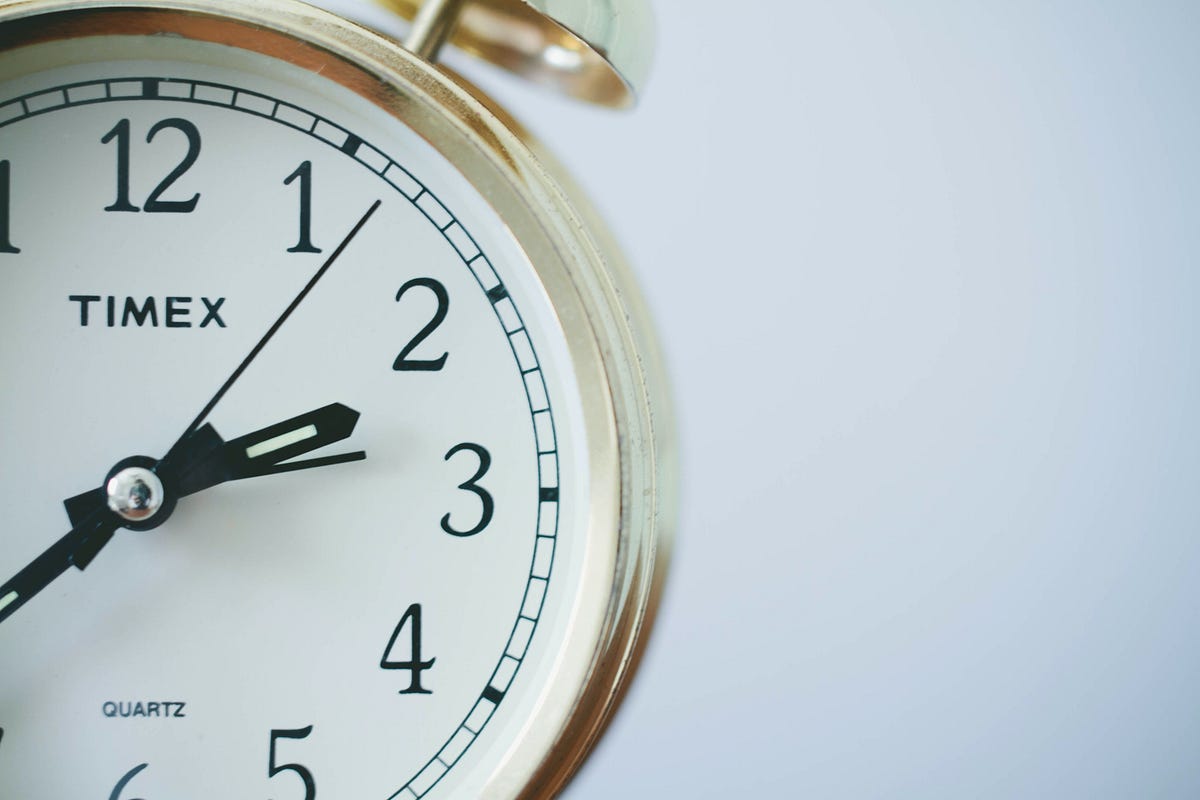
https://www.gartner.com/en/documents/5532895
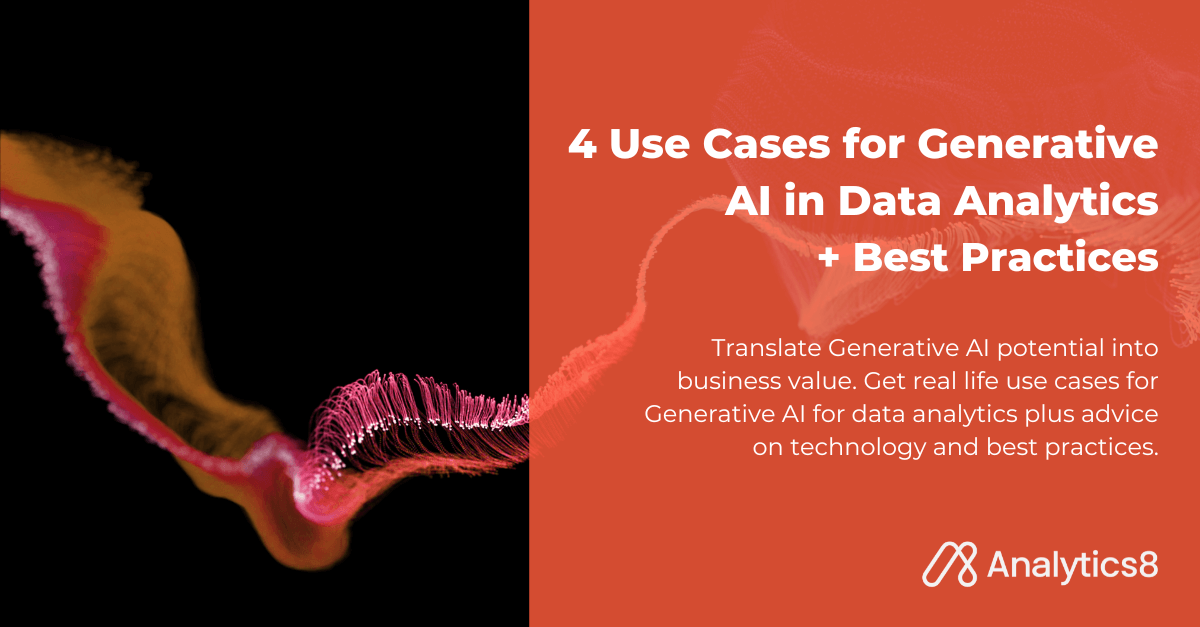
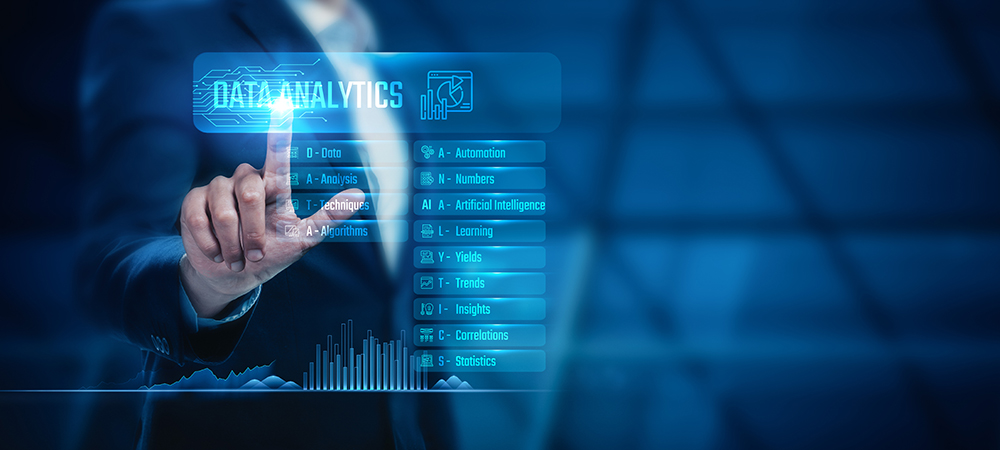
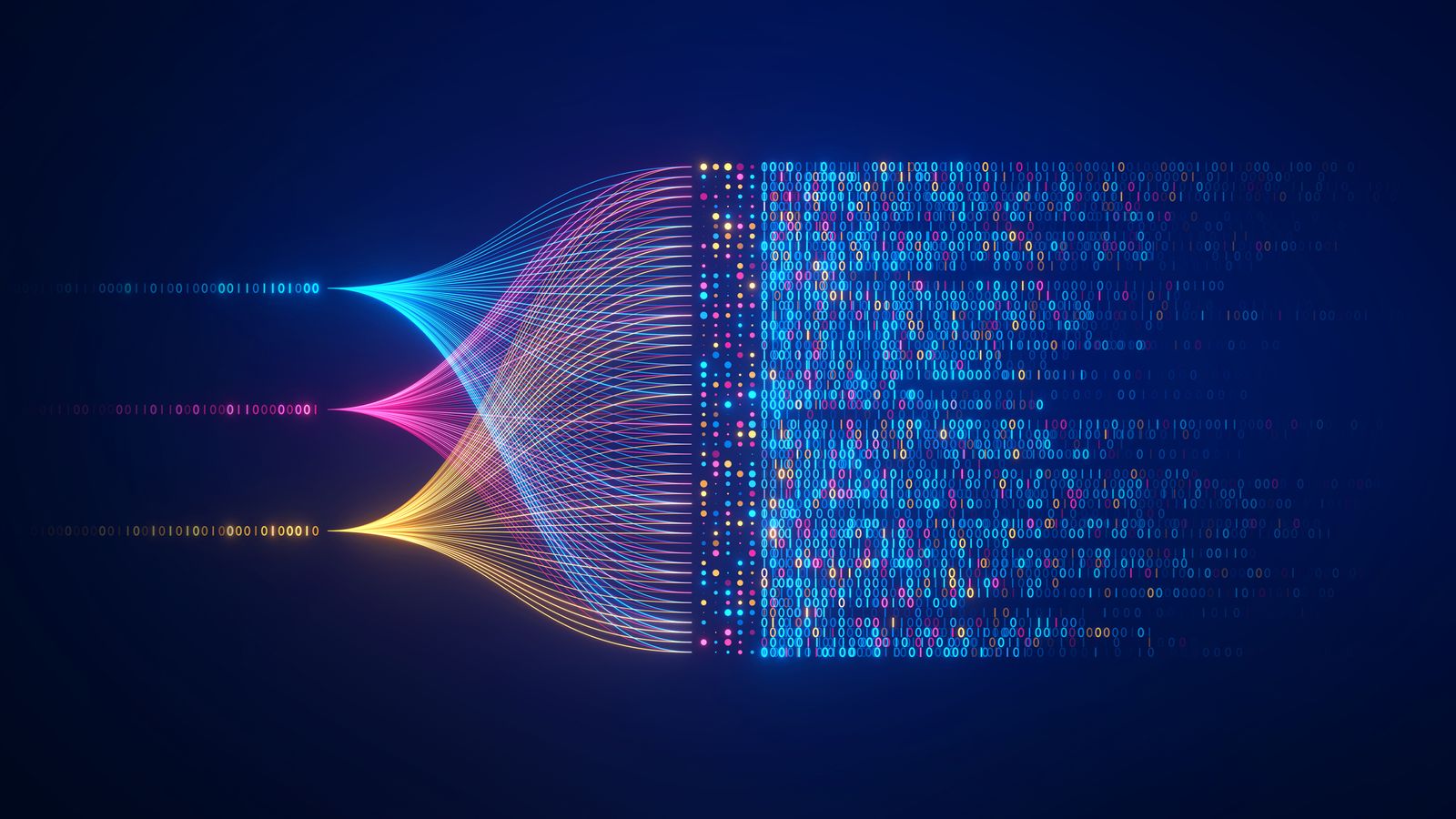
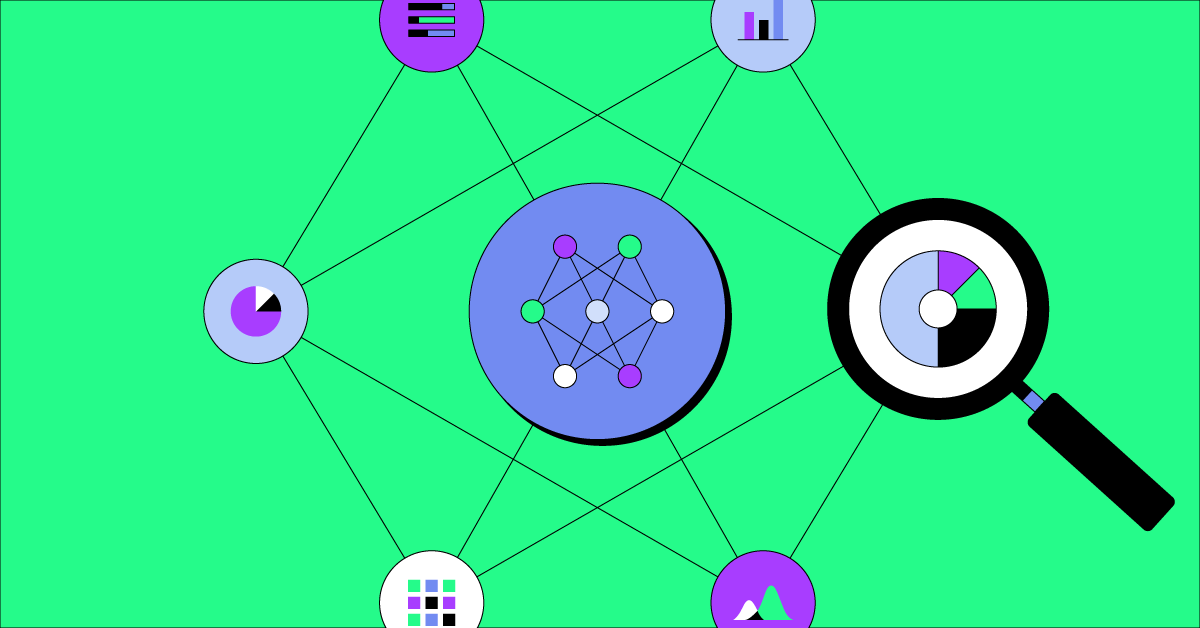
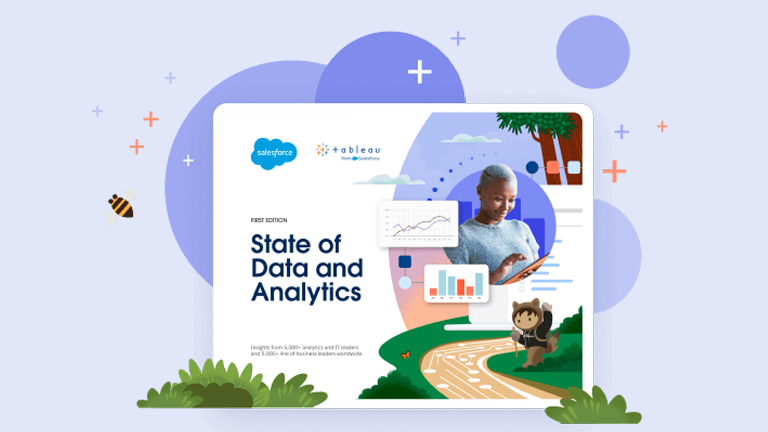